Problem Statement & Introduction
Have you ever wondered how Intelligent Tagging (ITAG) – our custom-made Market Intelligence tool works? ITAG is designed to help intelligence professionals efficiently access and gain deeper insights from a wide range of sources, including news, blogs, and social media posts. One important feature of ITAG is document classification, which assigns documents to specific categories, such as news types, to serve as filters.
Currently, ITAG’s document classification is performed using rule-based models. While this approach has its advantages, such as quick implementation and easy-to-understand results with high precision, it becomes less compatible with deployment on a larger scale as the number of ITAG projects increases. To improve scalability and performance of the ITAG tool, alternative approaches such as advanced machine learning-based models are being considered.
Last year, we investigated the application of unsupervised learning models, specifically topic models, for the task of categorizing documents within a corpus. Using unsupervised models for this task has various benefits, particularly when compared to supervised classification methods. One of the key advantages is that manual annotation of text with labels is not required, resulting in a significant reduction of time and effort. Additionally, it is not necessary to define categories beforehand as the models are able to discover and identify them automatically.
The research was conducted on the ITAG data of a client that offers technological solutions to classical music professionals. The aim of this ITAG is to provide valuable news and insights related to the classical music industry, such as event updates, featured artists, and other industry-related information. The data is multilingual, with a majority of articles written in English and French. Specifically, this study focused on categorizing news articles using topic modeling techniques. The former categorization method contained the following predetermined categories such as Competition, Festival, Release, Premiere, Review, Education, Interview, and Other. The study’s goal was to determine whether topic modeling can effectively categorize articles within these predefined categories and identify new ones, while also exploring the potential for improving scalability and performance of the ITAG tool.
Unsupervised models can be difficult to evaluate, so we will also analyze the 20 newsgroups dataset, which is publicly available. This dataset contains articles that are labeled with their corresponding newsgroups, and can serve as a reference for assessing the performance of our categorization techniques. In the science category, there are specific topics such as electronics, cryptography, medicine, and space. In our analysis, we will only consider the scientific articles, which include the four specific topics mentioned and consists of 2’361 documents, as the typical ITAG projects involve datasets that are specific to a particular industry.
Theory
Topic modeling is a technique used in machine learning to discover abstract themes within a large collection of unlabeled documents. In our research, we focused on two models, Latent Dirichlet Allocation (LDA) and BERTopic. LDA [1] is a probabilistic model that assumes each document can be represented as a mixture of latent topics, while BERTopic [2] is a recently developed technique that combines state-of-the-art machine learning algorithms to identify topics. One limitation of LDA is that it uses a bag-of-words representation of text, which does not take into account the semantic relationships among words. BERTopic addresses this issue by representing text using pre-trained contextual embeddings that capture the contextual nature of the text. In this post, we particularly explored the process of using BERTopic for topic modeling.
BERTopic is a three-step process that begins with generating document embeddings using pre-trained language models. These embeddings represent the abstract meaning and relationships of words, and are able to capture the semantics of the text. BERTopic uses Sentence-BERT [3], an extension of BERT that produces semantically meaningful sentence embeddings using siamese and triplet network structures. BERTopic supports several transformer embedding models, including multilingual models, making it a versatile technique for topic modelling. The next step involves reducing the dimensionality of these high-dimensional vectors using the UMAP method [4], which is particularly effective at preserving the local and global features of high-dimensional data.
BERTopic then uses an unsupervised machine learning technique called clustering to group these documents into clusters based on their similarity using HDBSCAN algorithm [5] which is able to identify clusters of varying density in large and complex datasets. The final step of BERTopic involves extracting keywords from each cluster using a modified version of TF-IDF called class-based TF-IDF [6], which calculates term frequency and inverse document frequency at the cluster level, allowing for a better understanding of the main topics discussed within each cluster.
Methodology
The methodology used in this study consists of five steps: Document Filtering, Text Preprocessing, Hyperparameter Selection for Topic Models, Evaluation, and Mapping Topics to Categories. Both LDA and BERTopic models followed a similar order of these steps, with minor variations depending on the model. To differentiate between the two, we will refer to the approach using the LDA model as the bag-of-words approach, and the approach using the BERTopic model as the language model approach.
- Document Filtering: The first step is to filter out near-duplicate documents [7], which are almost identical pieces with minor differences. Additionally, event detection is performed to identify articles related to the same event and prevent over-representation [8][9]. Filtering out near-duplicates and event-related articles helps improve the quality of the resulting topics.
- Text Preprocessing: Preprocessing steps vary depending on the approach used. For the Bag-of-Words approach, preprocessing involves removing punctuation and numbers, lowercasing, lemmatization, stopword removal, and infrequent term removal. In the Language Model approach, less preprocessing is required to maintain the original sentence structure [10].
- Hyperparameter Selection for Topic Models: Adjusting hyperparameters is essential to ensure high-quality results. For LDA, the number of latent topics and the α and β parameters are adjusted. The number of topics determines the granularity of topics, while α and β control the distribution of topics within documents and words within topics, respectively. For BERTopic, hyperparameters in the UMAP and HDBSCAN stages, such as the number of neighboring sample points and the minimum cluster size, are selected.
- Evaluation: Evaluation of topic models focuses on interpretability. Topic Coherence, Topic Diversity, and Clustering Evaluation are used as evaluation metrics. Topic Coherence measures the semantic similarity of high-ranking words within a topic, while Topic Diversity ensures distinct and comprehensive coverage. Clustering Evaluation compares the output clusters with labels using metrics such as Purity and Normalized Mutual Information.
- Mapping Topics to Categories: After selecting the optimal topic model, topics are assigned to specific categories based on human judgment and visualization techniques. This step ensures consistent and accurate categorization, with visualization aiding in understanding the topic structure.
By following these steps, the study aims to uncover meaningful and diverse topics while maintaining interpretability and alignment with domain knowledge.
Results
The results highlight the trade-offs between Overall Quality (product of Topic Coherence and Topic Diversity) and NMI scores, as well as the challenges of assigning categories in topic modeling.
They are divided into two parts: one applied to a reference dataset and another to a classical music dataset.
- Science reference dataset
- Bag-of-Words Approach: Near-duplicate detection resulted in 7 communities, and after filtering, 2,352 unique documents remained. LDA models were trained with different hyperparameter combinations (K ranged from 2 to 8 with a step size of 2, and α and β, took values of 0.1, 0.25, and 0.5), resulting in 36 models. Evaluation based on Overall Quality and NMI scores revealed the top models. Interestingly, the second-best model based on Overall Quality resulted with 4 topics but had a low NMI score, indicating a discrepancy. The best model according to Overall Quality had 8 topics but lacked specificity.
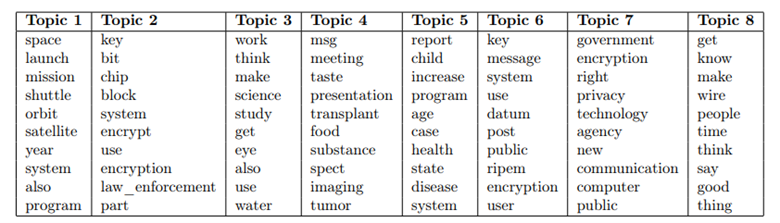
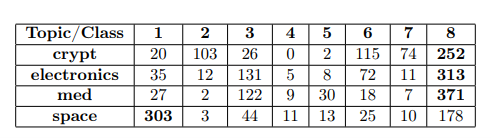
It seems that, Topic 1 could describe “space” articles, Topic 2 and 7 could represent “crypt” articles, Topic 4 and 5 could be about “med”, Topic 6 can describe “electronics” and “crypt”. However, the results from cross-intersections don’t always agree with these mappings. For example, Topic 4 contains more “space” articles than “med” articles. From the analysis of Table 3, it can be determined that the majority of documents labeled as “crypt,” “electronics,” and “med” are found in Topic 8. This topic groups the most common words, which primarily consist of verbs and do not represent any specific group. Additionally, Topic 3 also extracts common words without representing a specific group. However, it can be observed that there are high values for intersection in certain cases. For example, Topic 2, 6, and 7 effectively represent the “crypt” class, and Topic 6 appears to contain many “electronics” articles. Notably, only documents labeled as “space” are found in majority in a separate topic – Topic 1, which effectively captures the terms that characterize these documents. Overall, while the model demonstrates some limitations in mapping topics to classes, the top words per topic may provide valuable insights about the composition of the corpus.
The optimal model based on NMI had 4 topics and effectively identified the classes “space” and “crypt,” with extracted top words and a high degree of accuracy for cross-intersections, it also managed to produce a topic with the top words related to topic “med”, however the majority of “med” articles were not mapped to that topic. The class “electronics” was not mapped to any particular topic.
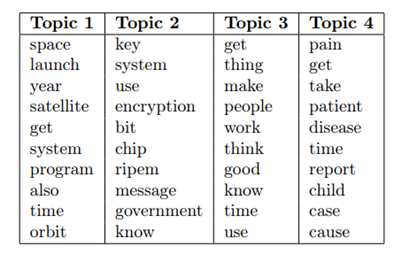
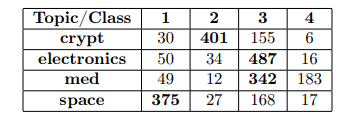
- Language Model Approach: Near-duplicate detection identified 10 communities, and after filtering, 2,301 documents remained. UMAP visualizations (in 2 and 3 dimensions) with n_neighbors set to 15 showed coherent and 4 well separated clusters. Below, we see the reduced embeddings in 2 dimensions.
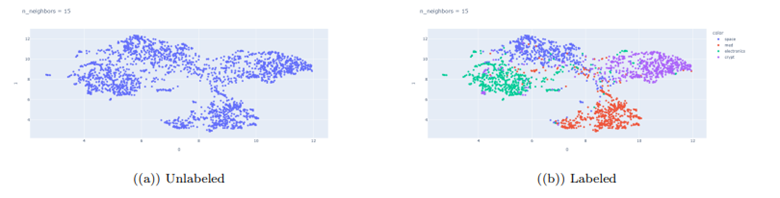
To select the HDBSCAN hyperparameters, we conducted a search for the optimal min_cluster_size and min_samples parameters generating 45 model combinations. Out of 45 combinations, 33 identified that the dataset contains 4 topics, indicating that the majority of the models were able to successfully identify stable topics. The model with the highest NMI and the highest Overall Quality score both resulted in clustering with 4 topics. The model with the highest NMI score performed better in mapping and identifying top words, while the Overall Quality model had more outliers but managed to capture well the essence of the 4 topics.
Below we present the top words and the cross-intersection tables generated by the model with the highest Overall Quality score. Topic -1 corresponds to outliers/undetected documents.
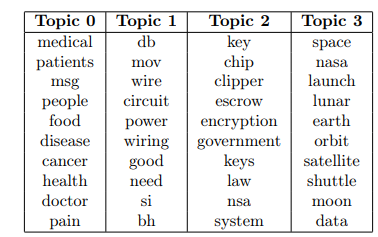
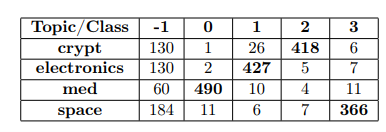
We can also see the reduced embeddings that are presented and color-coded by the extracted topics. Additionally, the figure on the right illustrates the classification of the assignments, with correctly assigned instances represented in green, incorrectly assigned instances represented in red, and outliers represented in blue.
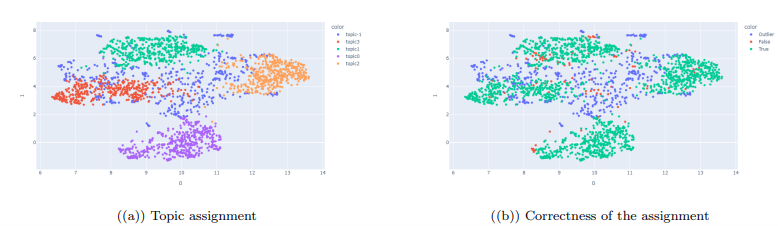
2. Classical Music Dataset:
- Bag-of-Words Approach: Near-duplicate detection identified 398 communities, and after filtering, 6,072 articles remained. LDA models were trained with various parameters, and the best-performing model had 20 topics. Categories were assigned based on dominant topics, topics such as Concerts and Reviews were identified, but some topics were challenging to assign. Several topics could be mapped to news about artists. We also identified a topic which describes articles about the Ukrainian and Russian war, which is an outlier as the news is not related to classical music. The rest of extracted topics were difficult to map to a specific category as they regroup common words across all documents
- Language Model Approach: After filtering, 5,698 documents remained. The chosen parameters for UMAP and HDBSCAN in BERTopic were determined through exploration of keywords and evaluation scores of 105 models with multiple combinations of hyperparameters. The best model based on the Overall Quality score generated 8 topics but lacked quality. Therefore, the model with 20 topics was chosen.
Upon further examination of the articles mapped to specific topics, we managed to find topics linked to the Education, Reviews, Competitions, Festivals, separate topics for Concerts in different genres. Furthermore, we have identified certain categories that were not predefined beforehand such as topics that can be mapped to political news, news about the Royal English family, news about Russian artists who have been sanctioned due to their political views, news about Death Announcements, Visual Arts news, Dancing shows news, and Theater show news.
Conclusion
The task of unsupervised categorization of unlabeled documents can be quite challenging, particularly due to the presence of outliers and underrepresented categories. Despite these challenges, both Latent Dirichlet Allocation (LDA) and BERTopic were effective in identifying various categories of interest within the collected news articles. Notably, BERTopic outperformed LDA in terms of flexibility and efficiency, benefiting from its use of advanced language models, an efficient clustering stage, and the automatic detection of the number of topics. This was especially evident in the reference dataset. The employed methodology in this study, which prioritizes avoiding the grouping of documents into events rather than topics, also contributes to more satisfying final results.
The categorization task could be improved by integrating unsupervised learning with supervised methods. Specifically, topic modeling, especially BERTopic, can be utilized in the initial stage to identify categories of interest and gather high-quality data. Also, re-sampling techniques can be employed to achieve a better distribution of data across categories.
This study was conducted and presented to the University of Geneva by Daryna Bilodid.
Resources:
[1] David M Blei, Andrew Y Ng, and Michael I Jordan. Latent dirichlet allocation. Journal of machine learning research, 3, 2003.
[2] Maarten Grootendorst. Bertopic: Leveraging bert and c-tf-idf to create easily interpretable topics. Zenodo, Version v0, 9, 2020.
[3] Nils Reimers and Iryna Gurevych. Sentence-bert: Sentence embeddings using siamese bert-networks. arXiv preprint arXiv:1908.10084, 2019.
[4] Leland McInnes, John Healy, and James Melville. Umap: Uniform manifold approximation and projection for dimension reduction. arXiv preprint arXiv:1802.03426, 2018.
[5] McInnes, John Healy, and Steve Astels. hdbscan: Hierarchical density based clustering. J. Open Source Softw., 2, 2017.
[6] Thorsten Joachims. A probabilistic analysis of the rocchio algorithm with tfidf for text categorization. Technical report, 1996.
[7] Alexandra Schofield, Laure Thompson, and David Mimno. Quantifying the effects of text duplication on semantic models. In Proceedings of the 2017 conference on empirical methods in natural language processing, 2017.
[8] Andrei Z Broder. On the resemblance and containment of documents. In Proceedings. Compression and Complexity of SEQUENCES 1997 (Cat. No. 97TB100171), pages 21–29. IEEE, 1997.
[9] Charu C Aggarwal and Karthik Subbian. Event detection in social streams. In Proceedings of the 2012 SIAM international conference on data mining, pages 624–635. SIAM, 2012.
[10] Jordan Boyd-Graber, David Mimno, and David Newman. Care and feeding of topic models: Problems, diagnostics, and improvements. Handbook of mixed membership models and their applications, 225255, 2014.