Imagine a future in which AI actively participates in real-time knowledge acquisition in addition to crafting replies. Beyond Generative AI’s traditional content production capability, RAG (Retrieval Augmented Generation) offers a new future in which machines provide both creativity and information accuracy.
In this blog post, you will discover the concept of Retrieval Augmented Generation through the example of chatbots, such as ChatGPT, and what this means for Artificial Intelligence.
Large Language Models
The core of chatbots
Most of us surely have encountered chatbots or virtual assistants that imitate human conversation. In certain circumstances, it’s difficult to tell if we’re talking to another human or a machine. Large Language Models have the ability to interpret and imitate human language, allowing consumers to engage with ChatGPT or other chatbots without any coding or computing abilities.
To put it more technically, Large language Models (LLMs) are a type of Deep Learning model specialised in processing and generating human textual language. The model improves through training on a huge set of text data. As a result, LLMs can perform a wide range of language-related tasks, including translation, dialogue generation, responding to questions, information extraction and summarisation, and even creative writing, among others.
Limitations
Despite the ability of LLMs to mimic human communication patterns through the use of proper syntax and grammar, they fall short in grasping real-world context. Additionally, LLMs struggle significantly with retrieving and manipulating their stored knowledge. This deficiency manifests in several critical issues, including the generation of incorrect information (known as hallucination) and a lack of proficiency in specialized domains.
If you’d like to know more about how LLMs work and their real use cases, read more on our previous blog posts Unleashing the Power of LLMs: An Effixis Guide Part 01 & Part 02.
How can we minimize hallucinations and ensure the information provided is high quality and factually accurate? This overview explores a well-regarded technique for enhancing the knowledge base of a LLM – retrieval-augmented generation (RAG). This strategy is crucial for incorporating real-world knowledge into LLMs, with the goal of improving the reliability and accuracy of the information they produce.
RAG: potential solution to LLMs limitations
Retrieval Augmented Generation (RAG) is a technique designed to address the limitations of LLMs. RAG integrates data from external sources, such as web or internal databases. The goal is to refine the inputs for LLMs, ensuring that they provide users with relevant and accurate responses. This involves enhancing the models’ understanding by incorporating additional context into the inputs. By doing so, we aim to improve the LLMs’ ability to interpret and respond to user requests more effectively, leveraging context to guide the models towards more precise and contextually appropriate answers.
RAG provides a source of real-time information that would be otherwise challenging to obtain from an LLM because continuous training is expensive and not feasible. By integrating a retrieval mechanism, LLMs can access up-to-date information from a wide range of sources in real-time. This approach significantly enhances the model’s ability to generate relevant responses by leveraging the latest information available, which is especially beneficial for questions that require current data.
How does Retrieval Augmented Generation (RAG) work?
RAG pipelines utilize advanced methods, often semantic search and vector databases. For those interested in a more detailed technical discussion, we recommend checking out our other blog post.
While there isn’t a single method for retrieving relevant information within the LLM workflow, we’ll break down a basic document-retrieval pipeline in simple terms for better understanding.
The data and text files, which provide the knowledge base for the LLM, are divided into numerous segments. These segments are then vectorized, meaning they are converted into vectors (i.e., they are represented as numerical values in a multidimensional coordinate system). These vectors essentially capture the semantic essence of each segment. Consequently, when a query is sent by the user, it is also converted into a vector. The system identifies the relevant information by finding text segments whose vectors are semantically close (in terms of distance) to the vector of the query. This process enables the precise retrieval of information that matches the query’s context.
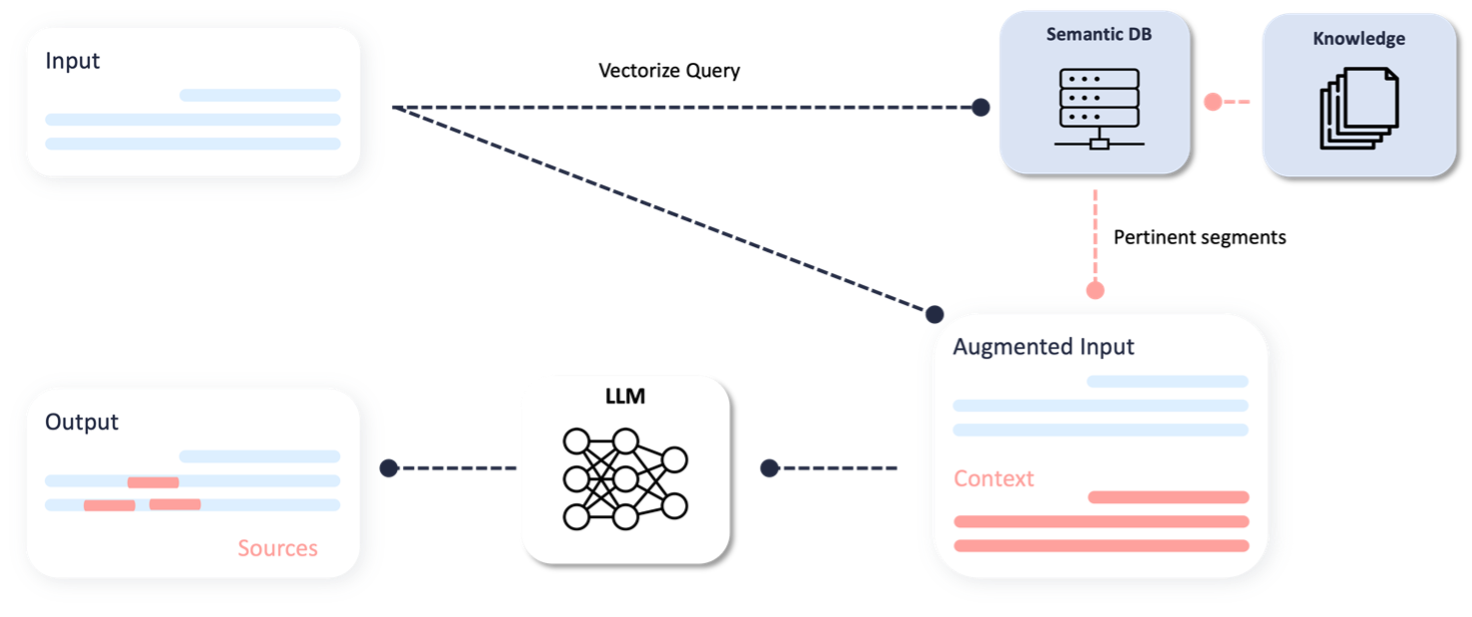
Retrieval Augmented Generation’s Impact on Ethical AI concerns
In addition to a gain in reliability and accuracy, the use of RAG addresses a number of concerns around the ethical use of Artificial Intelligence.
Training large AI models demands considerable electricity, computational resources, and time. By eliminating the necessity for additional rounds of intensive computational training, we could aim to pursue a more environmentally sustainable path for Artificial Intelligence.
By gathering reliable and contextually relevant information, RAG makes a substantial contribution to the fight against fake information propagation. Its capacity to obtain trustworthy data contributes to the promotion of credible, fact-based material, which in turn promotes a better-informed public.
Through the utilisation of many sources, RAG also possesses the capability to reduce biases present in particular datasets. It can offer a more impartial perspective by compiling data from various sources and points of view.
Final Considerations
While RAG, like Generative AI, has several benefits, it is not without challenges. The most important of them is the fundamental need for transparency in data sources.
Systems that use RAG should be carefully built to cite the source of their information, whether it is a text, a web page, or a video. This degree of clarity, which we maintain in partnership with our customers across a wide range of industries including pharmaceuticals, legal, medical, and consumer goods, allows users to easily cross-check the information.
There is also a learning curve, and adopting it may need extensive training, particularly in prompt engineering, in order to take full advantage of its possibilities. Expertise in Prompt Engineering is quickly becoming more important when interacting with various AI models, but especially conversational ones like ChatGPT. To meet this growing demand, Prompt University is stepping forward, offering dedicated training in Generative AI and Prompt Engineering.
However, beyond the surface-level challenges lies the critical aspect of data sourcing. The success of RAG also relies on the data behind it. The data has to contain the information allowing to answer the question and in an easily retrievable structure. This underscores the necessity of a robust data preparation phase, encompassing meticulous data cleaning and chunking (i.e., dividing a document into smaller pieces).
These preparatory steps are important for ensuring that the retrieval process is both efficient and effective. Without a well-architected approach to data indexing and retrieval, the performance of the RAG system could be compromised. Thus, it is crucial to address these challenges head-on, ensuring that the data pipeline is finely tuned to support the operation of Retrieval Augmented Generation systems.
In summary, RAG emerges as a promising solution to the limitations faced by LLMs. With careful refinement and continued innovation, it has the potential to transform the landscape of Generative AI by providing a route towards more informed, credible, and ethical AI-driven interactions.
Contact us at contact@effixis.be to learn more about RAG and its applications adapted to your unique circumstances.
Sources
[1] The Environmental Cost of LLMs: A Call for Efficiency
[2] Retrieval Augmented Generation Tools: Pros and Cons Breakdown
[3] IBM Technology: What is Retrieval-Augmented Generation (RAG)?
[4] Databricks: What challenges does the retrieval augmented generation approach solve?